Forecasting the future
- September 2, 2021
- Posted by: eknkln
- Categories: Business Analytics, Finance & Accounting, Strategic Management
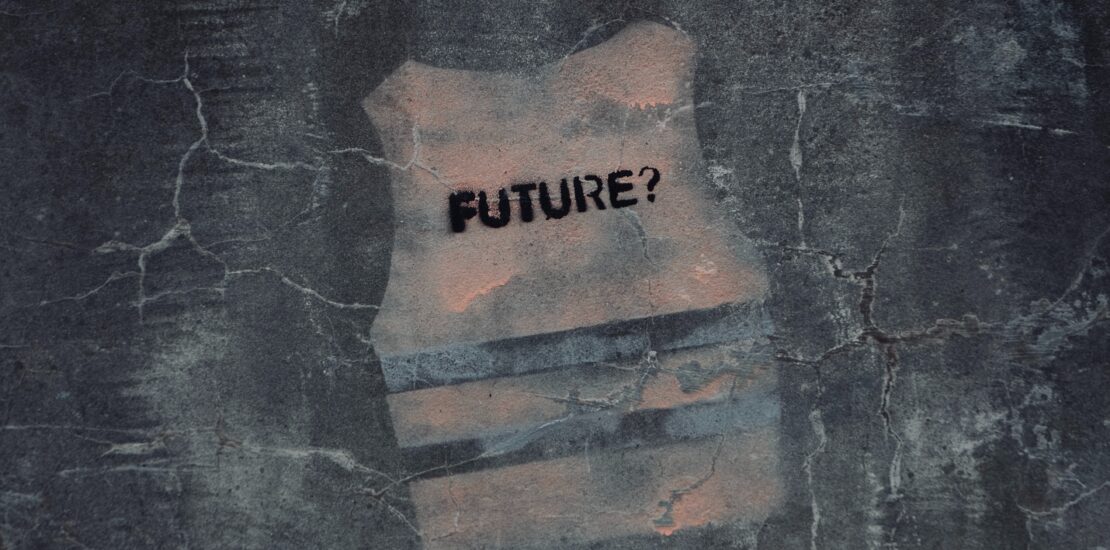
Easily the biggest financial planning and analysis (FP&A) challenge for most companies is predicting future revenues and cash flows—a challenge that became much harder during the COVID-19 pandemic. Past results and old assumptions may no longer be sufficient, and new market factors need to be considered. How can we prepare for different scenarios?
WHAT IS PREDICTIVE ANALYTICS?
SAS defines predictive analytics as “the use of data, statistical algorithms and machine learning techniques to identify the likelihood of future outcomes based on historical data,” noting that the goal is to go beyond knowing what has happened to decrease uncertainty about the future and associated risk (see “Predictive Analytics: What it is and why it matters,” SAS Insights). Predictive analytics can be used to get clarity on what the future can look like. In its simplest form, it’s the science (and a little art) of taking historical data and using it to project future results.
Keep in mind that the ultimate goal of forecasting is to prepare for future outcomes by reducing uncertainty and associated risk. Outcomes could include a new product or service taking off much higher or lower than expected. Risks include not having enough capacity to meet demand, missing market opportunities altogether, overinvesting in new assets such as people and equipment, and so forth. Thus, any information that can be potentially useful to reduce uncertainty can add value to predictive models.
FIVE WAYS TO USE PREDICTIVE ANALYTICS
Effective predictive analytics needs data, tools, model building, and skills. The SMA presents nine ways to facilitate effective predictive analytics. In this article, we briefly describe five of them.
1. Expand the data available.
Good predictive analytics requires that a wide range of data be available. As a management accountant, you not only need to be able to expand the information available for the finance department, but you may also need to expand your view of which data is important for forecasting purposes—and you may even need to identify and collect that data. You can help build a “data lake” that holds a vast amount of data in its native format until it’s needed for management purposes. The data lake should support a wide range of analytics. Table 1 provides various sources of data within organizations.

A common error committed by forecasters is considering only factors and data that are internal to the organization. Factors external to the organization must also be considered. The world is interlinked and globalized. What happens in China affects what happens in Baltimore, Md., in the United States.
But this can make forecasting more difficult. How do you account for these factors? What if you miss a few, and how do you know which are important? Strategy planning is key. Before any forecasting is done, the strategic planning process should include an assessment of external factors and plans for addressing them. Thus, the forecasting model should capture the most important of these external factors.
2. Tools: Start small and add on.
You may be thinking that predictive models would have to be massive to capture all the key drivers in an organization, and many are. You may also be thinking of your already massive Excel workbook, and the thought of adding more types of data along with how different drivers are related makes you start to think about retiring. The good news is that predictive models should start small to include the most significant variables and approximate relationships among the variables.
Once you establish a base model that you’re satisfied includes all the major variables, start adding variables to increase its accuracy. When the model gets sufficiently complex to make updating key assumptions cumbersome, it’s probably time to find one of many FP&A software solutions available.
Don’t try to build a model that includes everything about your company. First, that’s impossible. Second, it isn’t worthwhile. The key is to think long-term accuracy over short-term precision. By design, building predictive models is meant to reduce uncertainty about the future. Because the future is uncertain, it isn’t worth trying to accurately model relatively low-impact drivers.
3. Use scenario planning.
Scenario planning (also known as scenario analysis) is more than just listing several potential scenarios. In fact, it isn’t even possible to list all of the potential scenarios, let alone predict them. Instead, it’s meant to identify potential scenarios circling around one or two key focal issues, driving forces, or critical uncertainties. These might be the issues causing the biggest risks to the organization (e.g., cyber threats or customers not coming back) or a key driver of success (e.g., oil price on June 1, online traffic in May, or easing of COVID-19 restrictions).
For example, resorts, fairs, and beach towns have struggled with the uncertainty about pandemic restrictions and people’s desire to travel. Fireworks operators have been forced to provide clients with guarantees that if they’re forced to cancel an event due to COVID-19 restrictions, even at the last minute, clients will get their money back. That causes some significant and critical uncertainties for the fireworks operators. It takes up to a year to prepare for a big show. Will the frequency of cancellations be high or low? And if the scheduled shows do happen, will there be high crowd turnout or low crowd turnout, a leading indicator for the number of future events? Scenario planning can help by establishing different scenarios based on the most critical unknown variables that may occur and then estimating the likelihood of the different scenarios. In this example, the fireworks operator might envision four scenarios using a 2×2 matrix as in Figure 1.
The planning team gives each scenario a name and composes a narrative as if it had already happened. This helps to articulate and estimate the probability of each scenario that can then be put into the model.
4. Address the knowing-doing gap.
There should be a strong connection between predictive analytics, competitive strategy, and operational execution. You may want to predict customer demand using sophisticated modeling techniques, but these predictions should be evaluated and understood in light of the planned strategic actions the company is taking to grow sales. Examples of these planned actions include investing in research and development to launch compelling new products, managing the life cycle around end-of-life for certain products, reprioritizing certain products in the portfolio and the marketing investment behind them, and doubling the number of sales associates. There should be performance measures linked to the planned actions and built into the predictive analytics model. Predictive analytics can and should assume that planned actions will occur.
What a company does or fails to do will have an impact on the business and might be the real explanation for why a predictive model “was wrong.” In fact, a common reason why the future doesn’t turn out as predicted is a failure to execute plans. Try not to overlook this point because it may be at the heart of the variances your organization experiences. Not considering this possibility can lead to “chasing rainbows” and fine-tuning models that will continue to fail us.
The knowing-doing gap is simply the gap between saying you’re going to do something and actually doing it. How do you know if a knowing-doing gap is undermining your ability to deliver valuable predictive models? A strong indication is if your organization consistently fails to meet its goals. The best answer is to combine predictive analytics thoughtfully by incorporating knowledge of the business and the company’s operational plans. Monitor progress on those plans too.
5. Model building: Think causality.
To build a reliable predictive analytics model, think causality. Causality is a function of operations: resources, processes, and activities. A reliable predictive analytics model should be based on actual or expected causal relationships among resources, processes, customers, key performance indicators, external market factors, and other leading or lagging measures. Remember to start small with the most critical causal factors and, once validated, add variables as warranted to improve predictive value.
The process for developing a causal-based model is similar to developing a balanced scorecard. There should be a chain of cause and effect, leading and lagging indicators ultimately linked to desired financial outcomes. In fact, companies that have constructed such a scorecard have essentially designed a predictive model. If designed well, it should represent the company’s theory on which activities it needs to do really well on to ultimately be successful. Table 2 provides examples of leading and lagging factors that might be used in sales forecast models.

One way to determine key drivers is to start by asking straightforward questions about how your resources and processes function under various demand scenarios. Going back to the uncertainty in travel and resorts, for instance, a beach hotel might ask questions like, “How many lifeguards do we strive to maintain based on the number of tourists?” or “How does housekeeping cost increase based on tourist volume?” Look for explanations such as, “We maintain at least three lifeguards when the beach is open and one more when we hit 50% occupancy, one more at 75% occupancy, and one more at 100% occupancy.” And “We have a minimum of two housekeepers working eight-hour shifts, and then at about 50% occupancy, we increase housekeeping hours about one hour per every two rooms occupied.” Of course, to be able to ask the right questions and understand the explanations, it’s essential to understand the business model, who the customers are and where they come from, what value the company provides them, who does what, etc. If you don’t know, ask questions until you do.
Other variables needed for a complete model include those related to external factors. What is the competition doing? What are customers doing? What’s happening in the global environment? Even though these factors are essentially beyond the company’s control, they must be included in some way if they can significantly impact the business.
That was a lot to ask for before the pandemic, and in the post-COVID marketplace uncertainty, there may be totally new questions to ask. The business model has probably changed, and the data needed to succeed may have changed as well. New questions might include: How will COVID-19 restrictions impact our volume? How about social media and trade wars? What do we learn from call center data?
Once you have logical explanations and reasonable assumptions for various relationships, you’re ready to start building (or adding to) a model. One risk to be aware of is “model creep,” where the model (or accompanying spreadsheet) gets too complex to understand or maintain. Model builders tend to have a complexity bias toward complicated rather than simple. The precision necessary depends on the complexity of the question(s) being asked, but if one formula approximates the results of several formulas, use the one formula. Remember: The goal in forecasting is long-term accuracy (and understandability) over short-term precision.
FORECASTING IN ACTION
Every company has room for improvement in its forecasting, especially in today’s tumultuous markets and economies. The SMA discusses four additional ways to facilitate effective predictive analytics:
- Establish data collection systems.
- Improve assumptions and estimates.
- Monitor results and quickly identify the business reasons behind variances.
- Improve analytical skills.
Try not to feel overwhelmed if you or your finance team are lacking the time and skills needed to improve forecasting. Assess where you’re weakest and start there. Try a few of the steps mentioned here or in the SMA and build on that effort.
Forecasting the future is difficult but important. No matter how sound a forecast seems, reality will happen and often results will differ due to factors that simply couldn’t be foreseen logically. A good forecast supported with good planning can be a key tool for an organization to adapt rapidly to changes in assumptions, markets, and operations.
Make the decision to improve your forecasting
“Source : https://sfmagazine.com/post-entry/september-2021-forecasting-the-future/”